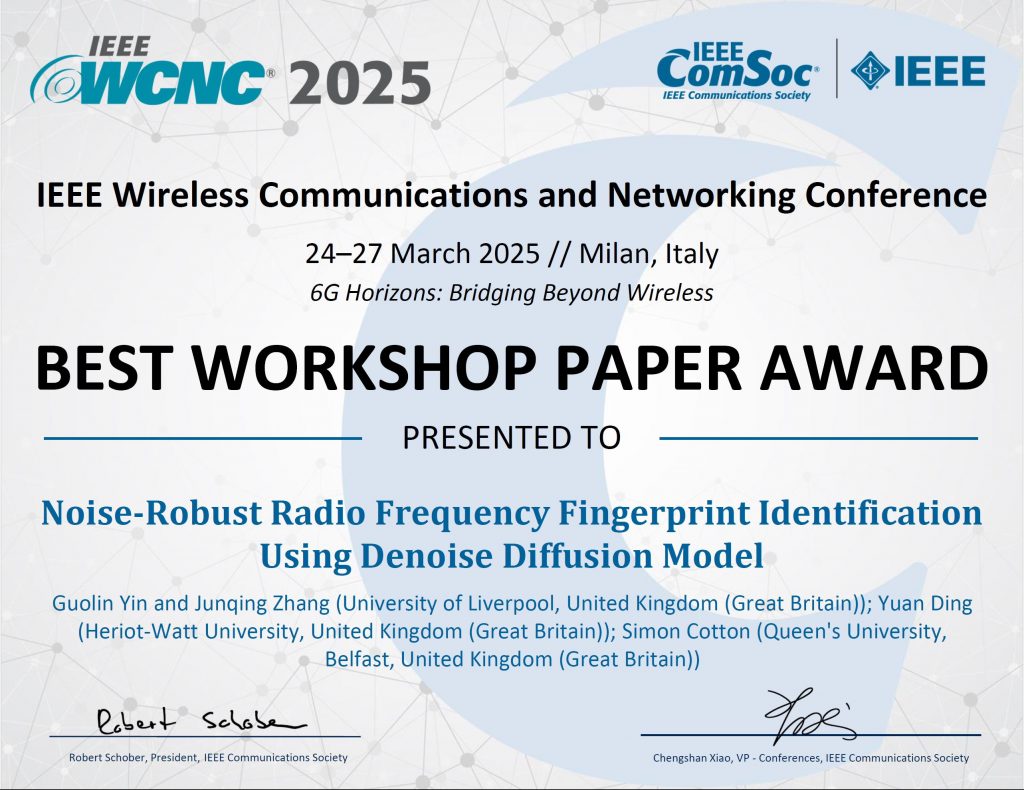
It is our honour that our paper ‘Noise-Robust Radio Frequency Fingerprint Identification Using Denoise Diffusion Model’ has been selected for the Best Workshop Paper Award in the IEEE Wireless Communications and Networking Conference (WCNC) 2025.
In this paper, we leveraged the diffusion model to effectively restore the RFF under low SNR scenarios. Specifically, we trained a powerful noise predictor and tailored a noise removal algorithm to effectively reduce the noise level in the received signal and restore the device fingerprints. We used Wi-Fi as a case study and created a testbed involving 6 commercial off-the-shelf Wi-Fi dongles and a USRP N210 software-defined radio (SDR) platform. We conducted experimental evaluations on various SNR scenarios. The experimental results show that the proposed algorithm can improve the classification accuracy by up to 34.9%.
This work is led by Dr Guolin Yin and Dr Junqing Zhang from University of Liverpool, and is supported by the Joint EPSRC project (University of Liverpool, Heriot-Watt University and Queens’ University of Belfast) – ‘Securing Spectrum Connectivity: Over-the-Air Authentication Using Radio Frequency Fingerprinting’.
Best Paper Awards | IEEE Wireless Communications and Networking Conference – IEEE WCNC 2025